FastApi-参数提交3
# 一、概述
一般对于Request Body不会通过get提交,对于get提交的参数一般称为是查询参数。所以,如果是通过POTS,PUT等方式提交的参数信息,我们一般是放到Request Body来提交到我们的后端。
对于如何接收和校验请求体,FastApi提供的形式是使用:from pydantic import BaseModel
示例如下:
import uvicorn
from fastapi import FastAPI
from pydantic import BaseModel
class Item(BaseModel):
name: str
description: str = None
price: float
tax: float = None
app = FastAPI()
@app.post("/items/")
async def create_item(item: Item):
return item
if __name__ == '__main__':
uvicorn.run(app='main:app', host="127.0.0.1", port=8000, reload=True, debug=True)
2
3
4
5
6
7
8
9
10
11
12
13
14
15
16
17
18
在上面的模型中,如果提交的Item它必须是怎么样的一个格式,比如name是必选字段,description是可选且默认为None, price是必选,且需要是float类型的,tax是可须且默认为None。
那客户端如何提交上面那些参数呐?
尝试提交参数什么都不写的情况下:
http://127.0.0.1:8000/items/
使用JSON格式提交参数的情况下:
{
"name":"Foo",
"description":"An openfdsf",
"price":45.4,
"tax":3.5
}
2
3
4
5
6
故意提交错误参数格式请求:
{
"name":"Foo",
"description":"An openfdsf",
"price":"45abc",
"tax":3.5
}
2
3
4
5
6
# Request Body 和 Query 和 Path的混合
在设计一些API过程中难免的可能也会需要综合遇到上述的一些混搭的组合,需要同时多个参数的提交和获取
那么我们通常接收这次参数的话一般怎么接收呐?
示例代码如:
import uvicorn
from fastapi import FastAPI, Path
from pydantic import BaseModel
app = FastAPI()
class Item(BaseModel):
name: str
description: str = None
price: float
tax: float = None
@app.put("/items/{item_id}")
async def update_item(
*,
item_id: int = Path(..., title="The ID of the item to get", ge=0, le=1000),
q: str = None,
item: Item = None,
):
results = {"item_id": item_id}
if q:
results.update({"q": q})
if item:
results.update({"item": item})
return results
if __name__ == '__main__':
uvicorn.run(app='main:app', host="127.0.0.1", port=8000, reload=True, debug=True)
2
3
4
5
6
7
8
9
10
11
12
13
14
15
16
17
18
19
20
21
22
23
24
25
26
27
28
通过之前的学习,其实也很简单道理也还是一样,如上的示例请求的话:
http://127.0.0.1:8000/items/1000?q=xiao
参数
{
"name":"Foo",
"description":"An openfdsf",
"price": 45.4,
"tax":3.5
}
2
3
4
5
6
# 多个Request Body的提交
更复杂的业务其实会存在多体的Boay的提交,之前做的商城下单里面,客户端有可能就会同时提交多个实体的对象信息到后端,如订单实体,地址实体,商品信息实体等。
那么在Fastapi如何接受多个Body实体呐?通常以前的话,在bottle,通常直接的request.body 或 request.json就可以获取客户端部提交的信息了。
在Fastapi假设客户端提交的参数是这样的形式:
{
"item": {
"name": "Foo",
"description": "The pretender",
"price": 42.0,
"tax": 3.2
},
"user": {
"username": "dave",
"full_name": "Dave Grohl"
}
}
2
3
4
5
6
7
8
9
10
11
12
那如何的接收处理呐?
import uvicorn
from fastapi import FastAPI, Path
from pydantic import BaseModel
app = FastAPI()
class Item(BaseModel):
name: str
description: str = None
price: float
tax: float = None
class User(BaseModel):
username: str
full_name: str = None
@app.put("/items/{item_id}")
async def update_item(*, item_id: int, item: Item, user: User):
results = {"item_id": item_id, "item": item, "user": user}
return results
if __name__ == '__main__':
uvicorn.run(app='main:app', host="127.0.0.1", port=8000, reload=True, debug=True)
2
3
4
5
6
7
8
9
10
11
12
13
14
15
16
17
18
19
20
21
22
23
这种情况,其实就是客户端提交多个实体对象。那可以定义多个模型对象即可。fastapi它会自动帮你处理提取信息。
http://127.0.0.1:8000/items/1000
如果另外再假设:
在Fastapi假设客户端提交的参数是这样的形式:
{
"item": {
"name": "Foo",
"description": "The pretender",
"price": 42.0,
"tax": 3.2
},
"user": {
"username": "dave",
"full_name": "Dave Grohl"
},
"importance": 5
}
2
3
4
5
6
7
8
9
10
11
12
13
其实这种可能也不是不存在滴,那如何的读取解析importance参数呐?既然参数有Query 和 Path,当然也会有 Body 。
import uvicorn
from fastapi import Body, FastAPI
from pydantic import BaseModel
app = FastAPI()
class Item(BaseModel):
name: str
description: str = None
price: float
tax: float = None
class User(BaseModel):
username: str
full_name: str = None
@app.put("/items/{item_id}")
async def update_item(
*, item_id: int, item: Item, user: User, importance: int = Body(..., gt=0)
):
results = {"item_id": item_id, "item": item, "user": user, "importance": importance}
return results
if __name__ == '__main__':
uvicorn.run(app='main:app', host="127.0.0.1", port=8000, reload=True, debug=True)
2
3
4
5
6
7
8
9
10
11
12
13
14
15
16
17
18
19
20
21
22
23
24
25
上面的代码中我们引入了Body 并且在importance: int = Body(...)进行处理和提取:
如果另外再假设,客户端提交的是一个单体对象内嵌的话,我们需要怎么处理?:
{
"item": {
"name": "Foo",
"description": "The pretender",
"price": 42.0,
"tax": 3.2
}
}
2
3
4
5
6
7
8
FastAPI提供了一个:
item: Item = Body(..., embed=True) 具体如下:
import uvicorn
from fastapi import Body, FastAPI
from pydantic import BaseModel
app = FastAPI()
class Item(BaseModel):
name: str
description: str = None
price: float
tax: float = None
@app.put("/items/{item_id}")
async def update_item(*, item_id: int, item: Item = Body(..., embed=True)):
results = {"item_id": item_id, "item": item}
return results
if __name__ == '__main__':
uvicorn.run(app='main:app', host="127.0.0.1", port=8000, reload=True, debug=True)
2
3
4
5
6
7
8
9
10
11
12
13
14
15
16
17
18
19
20
请求示例如:
如果另外再假设,客户端提交一个更复杂的嵌套模型的话,怎么办?麻蛋的 肯定也是会有这样的情况滴! 嵌套里面有列表有实体。比如:
{
"name": "Foo",
"description": "The pretender",
"price": 42.0,
"tax": 3.2,
"tags": ["rock", "metal", "bar"],
"image": {
"url": "http://example.com/baz.jpg",
"name": "The Foo live"
}
}
2
3
4
5
6
7
8
9
10
11
这时候,我们就需要所谓的子内嵌啦:
import uvicorn
from typing import Set
from fastapi import FastAPI
from pydantic import BaseModel
app = FastAPI()
class Image(BaseModel):
url: str
name: str
class Item(BaseModel):
name: str
description: str = None
price: float
tax: float = None
tags: Set[str] = []
image: Image = None
@app.put("/items/{item_id}")
async def update_item(*, item_id: int, item: Item):
results = {"item_id": item_id, "item": item}
return results
if __name__ == '__main__':
uvicorn.run(app='main:app', host="127.0.0.1", port=8000, reload=True, debug=True)
2
3
4
5
6
7
8
9
10
11
12
13
14
15
16
17
18
19
20
21
22
23
24
25
26
27
如上代码,Item里面包含了Image,也包含了,tags类型的列表定义。
MMP更深层的嵌套也是可以定义的如:
{
"name":"Foo",
"description":"The pretender",
"price":42,
"items":[
{
"name":"Foo",
"description":"The pretender",
"price":42,
"tax":3.2,
"tags":[
"rock",
"metal",
"bar"
],
"image":{
"url":"http://example.com/baz.jpg",
"name":"The Foo live"
}
},
{
"name":"Foo2",
"description":"The 2",
"price":422,
"tax":3.2,
"tags":[
"rock",
"metal",
"bar"
],
"image":{
"url":"http://example.com/baz.jpg",
"name":"The Foo live"
}
}
]
}
2
3
4
5
6
7
8
9
10
11
12
13
14
15
16
17
18
19
20
21
22
23
24
25
26
27
28
29
30
31
32
33
34
35
36
37
对应的解析为:
import uvicorn
from fastapi import FastAPI
from pydantic import BaseModel
from typing import List, Set
app = FastAPI()
class Image(BaseModel):
url: str
name: str
class Item(BaseModel):
name: str
description: str = None
price: float
tax: float = None
tags: Set[str] = []
# images: List[Image] = None
image: Image = None
class Offer(BaseModel):
name: str
description: str = None
price: float
items: List[Item]
@app.post("/offers/")
async def create_offer(*, offer: Offer):
return offer
if __name__ == '__main__':
uvicorn.run(app='main:app', host="127.0.0.1", port=8000, reload=True, debug=True)
2
3
4
5
6
7
8
9
10
11
12
13
14
15
16
17
18
19
20
21
22
23
24
25
26
27
28
29
30
31
32
33
请求url
http://127.0.0.1:8000/offers
# Request Body的Field
Field字段的意思其实就是类似上面Query, Path,也同样给Body内的字段的信息添加相关的校验。
也就是说。通过Field来规范提交的Body参数信息。比如:
import uvicorn
from fastapi import Body, FastAPI
from pydantic import BaseModel, Field
app = FastAPI()
class Item(BaseModel):
name: str
description: str = Field(None, title="标题啊", description="错误提示文字啊", max_length=30)
price: float = Field(..., gt=0, description="错误提示文字啊")
tax: float = None
@app.put("/items/{item_id}")
async def update_item(*, item_id: int, item: Item = Body(..., embed=True)):
results = {"item_id": item_id, "item": item}
return results
if __name__ == '__main__':
uvicorn.run(app='main:app', host="127.0.0.1", port=8000, reload=True, debug=True)
2
3
4
5
6
7
8
9
10
11
12
13
14
15
16
17
18
19
20
上面的意思就是和之前定义参数校验其实一样
正常情况:
{
"item":{
"name": "Foo",
"description": "The pretender",
"price": 42.0,
"tax": 3.2
}
}
2
3
4
5
6
7
8
9
异常情况:
{
"item":{
"name": "Foo",
"description": "The pretender sssssssssssssssss",
"price": 42.0,
"tax": 3.2
}
}
2
3
4
5
6
7
8
9
# 其他数据类型的校验
对于数据格式的校验,通常,我们不止于
int
float
str
bool
但是提交参数不止于上述的几种格式,有时候比如是对手机号码的校验,有些时候是时间类型的校验等
其他类型:
其他数据类型¶ 以下是您可以使用的一些其他数据类型(来自官方文档):
UUID:
一个标准的“通用唯一标识符”,在许多数据库和系统中常见于ID。
在请求和答复中,将表示为str.
datetime.datetime:
一只Pythondatetime.datetime.
在请求和答复中,将表示为str采用ISO 8601格式,如:2008-09-15T15:53:00+05:00.
datetime.date:
Pythondatetime.date.
在请求和答复中,将表示为str采用ISO 8601格式,如:2008-09-15.
datetime.time:
一只Pythondatetime.time.
在请求和答复中,将表示为str采用ISO 8601格式,如:14:23:55.003.
datetime.timedelta:
一只Pythondatetime.timedelta.
在请求和答复中,将表示为float总秒数。
Pydantic还允许将其表示为“ISO 8601时间差异编码”,有关更多信息,请参阅文档。.
frozenset:
在请求和答复中,将其视为set:
在请求中,将读取列表,消除重复,并将其转换为set.
在答复中,set将转换为list.
生成的架构将指定set值是唯一的(使用JSONSchema的uniqueItems).
bytes:
标准Pythonbytes.
在请求和答复中将被视为str.
生成的架构将指定它是str带着binary“格式”。
Decimal:
标准PythonDecimal.
在请求和响应中,处理方式与float.
所以我还可以使用其他类型来校验:
import uvicorn
from datetime import datetime, time, timedelta
from uuid import UUID
from fastapi import Body, FastAPI
app = FastAPI()
@app.put("/items/{item_id}")
async def read_items(
item_id: UUID,
start_datetime: datetime = Body(None),
end_datetime: datetime = Body(None),
repeat_at: time = Body(None),
process_after: timedelta = Body(None),
):
start_process = start_datetime + process_after
duration = end_datetime - start_process
return {
"item_id": item_id,
"start_datetime": start_datetime,
"end_datetime": end_datetime,
"repeat_at": repeat_at,
"process_after": process_after,
"start_process": start_process,
"duration": duration,
}
if __name__ == '__main__':
uvicorn.run(app='main:app', host="127.0.0.1", port=8000, reload=True, debug=True)
2
3
4
5
6
7
8
9
10
11
12
13
14
15
16
17
18
19
20
21
22
23
24
25
26
27
28
29
30
31
32
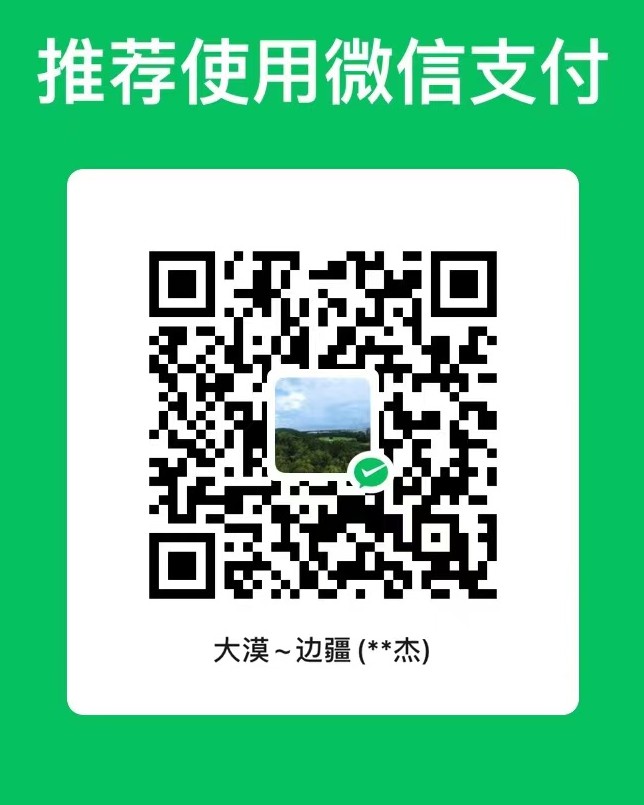
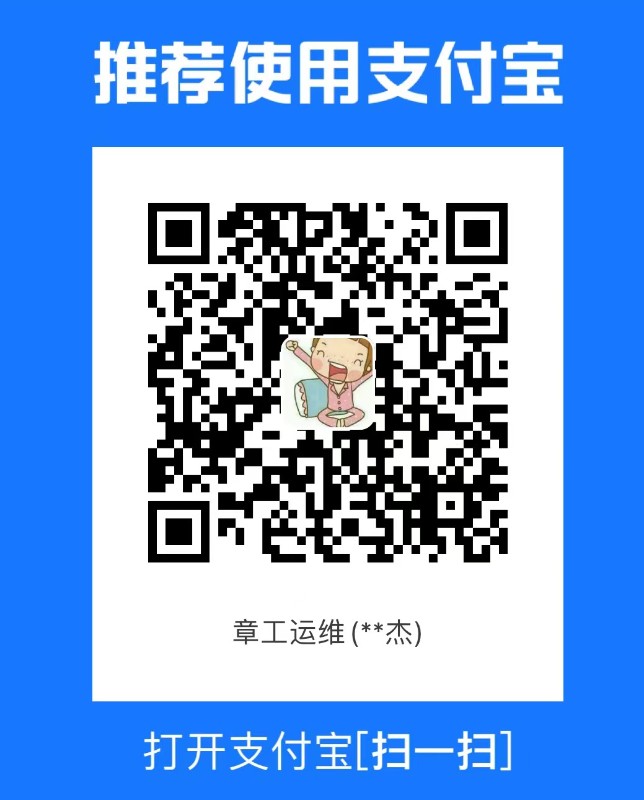